Announcing the latest version of Zelros open standard for a more responsible AI
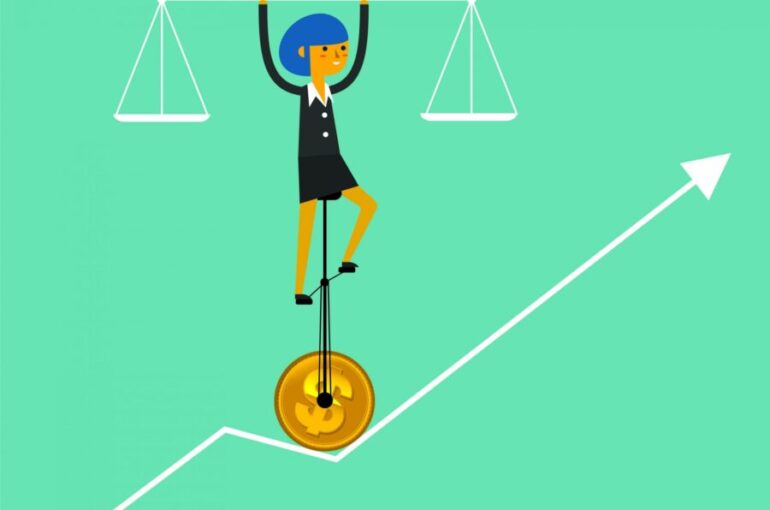
Exactly two years ago, we published a manifesto calling for an ethical use of AI, and introduced a dedicated open-source standard to document machine learning models deployed in production. We could not have imagined that, since that moment, this subject would have become so important. Indeed, to our great satisfaction, usage of fair and responsible AI has lately been attracting more and more attention.
Today, we are excited to announce a new update to this standard for a responsible AI. Here is why.
Biases in AI are not always where you think at first
Up to now, biases in AI have mainly been addressed through the lens of unbalance in training data. For example, when there are more men than women in the training dataset, or the other way around. This kind of bias is documented in section 5 of the standard: Training Data Audit.

However, unbalance in training data is not always synonymous with discrimination. For example, let’s take the case of an affinity insurer, particularly specialized in insurance for farmers, and mostly deployed in rural areas. When looking at the distribution of their client base, we will observe an under representation of social categories such as factory workers, or business executives. This could be seen at first as a bias, but in this case it is not a discriminative bias, it is a by design bias, because of the nature of the insurer.
Model biases versus data biases
To go further, another interesting question to ask, is to figure out whether AI models are working equally well over different sub-populations (sometimes called protected or sensitive groups). It is generally admitted that a machine learning model does not have 100% accuracy. This is assessed through internal tests called cross-validation, and typically documented in section 6.40 (Performance) of Zelros ethical AI standard report.

For example, let’s consider the previous insurer using an AI automated underwriting process to accept life insurance applications based on medical questionnaires, and having a 97% accuracy rate compared to manual decision. This could be considered as very accurate.
However, what about if we realize that the same AI model is actually working with 99% accuracy on farmers, and with only 79% accuracy on factory workers? This time, it could be considered as an unfair behavior, and it becomes important to document it.
Announcing the version 0.3 of Zelros standard for a more responsible AI
That’s why we are releasing today the version 0.3 of Zelros standard for a more responsible AI. This new version includes a new section 6.41, called Performance over protected groups, documenting the model performance over sub-populations.

Thanks to this new section auditors, regulators, governance officers, etc. … are now able to challenge the performance of any productized AI model, over sensitive sub-populations. For the first time, it becomes possible to detect new hidden, and up to now unknown, potential unfair behaviors.
For further details, you can access the full standard here.
What’s next
the version 0.3 of the standard is now fully supported by the Zelros platform, and allows to move again one step further towards a more responsible use of AI. Starting from now on, all models used by Zelros platform will support this new feature, contributing to a better algorithms transparency, which particularly important in the insurance sector.
Our research team is working on several further improvements, one in particular being the ability to mitigate detected model biases with synthetic data generation. Stay tuned for more announcements!