7 ways to foster a fair and responsible AI in insurance services
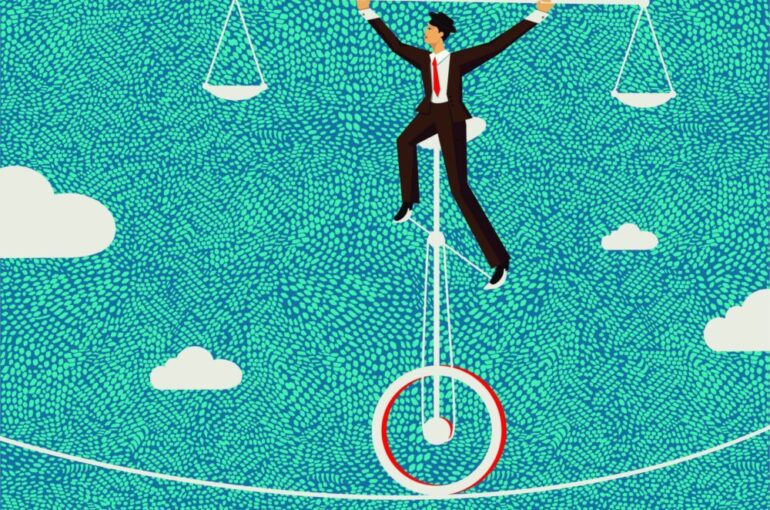
Since the creation of Zelros four years ago, we have witnessed tremendous progress in the use of machine learning and AI technologies all over the insurance value chain: distribution, customer service, claim, pricing, risk, …
Now that these new technologies start to be used at scale – and we are just at the beginning – we observe since a few months that the topics of fairness and trust are raising more and more attention. It is all the more a matter of concern as insurance is one of the industries which requires the most demands in terms of solidarity, fairness and equity.
This is something we are taking seriously at Zelros, and want to seize. This article shares our current thoughts on this subject matter, and random takeaways from the field.
Regulation
Why it matters?
What kind of AI do we want for our future? The answer is not straightforward. Recently, EIT Digital explored four anticipation scenarios (named dystopian, ultra-social, ultra-liberal or utopian), depending on regulation approaches (ranging from ‘soft’ to ‘firm’), and scope (from ‘context-dependant’ to ‘generic across-the-board’).
Being vigilant on this topic, and acting on pushing regulation in the right direction is an important mission to make a responsible, yet useful, use of AI.

What does it mean at Zelros?
The question of ruling AI is particularly important in the insurance and financial services sector, a traditionally highly regulated sector.
To remain up to date on this topic, and contribute to paving the way towards what could look like a regulation of insurance algorithms, we work closely with regulators. For example, we participated in the request for comments of ACPR (french regulator) regarding its recent guidelines “AI algorithm governance in financial sector”, and are involved at European level in the “Ethics guidelines for trustworthy AI”.
Education
Why it matters?
Don’t be naive: even with sophisticated regulation, protections and safeguards, it won’t be possible to totally avoid abuse in use of AI. The next level of defence against that, is education. It is crucial to raise citizens’ awareness on how data and machine learning work, so that they can develop their critical judgement, and are able to challenge suspicious usages of AI.
Some initiatives have already emerged, like for example the dedicated AI ethics online course from fast.ai.
What does it mean at Zelros?
Each year, we organize the Data Science Olympics, the largest Machine Learning competition in Europe, gathering 1000+ data fans in Paris and Berlin.
Of course, expert data scientists are participating. But we welcome also beginners, and coaches are available to initiate them to machine learning. By doing that, we try to help as many people as possible, to get a feeling of what AI is, and sharpen their knowledge about it.

Open Source
Why it matters?
Open sourcing is a very good way to share with the community, and to add transparency to AI initiatives.
Some forward-looking insurers already have a strong open source strategy, like for example MAIF who published its NLP engine automating policyholders inbound emails processing, and also its AI ethics maturity assessment framework.
What does it mean at Zelros?
18 months ago, we open sourced our Open Standard for Ethical Enterprise-Grade AI – a way of documenting machine learning models in production – and integrated it in our product.
Since then, similar approaches have been launched for example by Google (Model Cards) or IBM (Fact Sheets).
Tech
Why it matters?
Having the general objective of promoting a fair and transparent AI is a worthy purpose. But that being said, making it a technical reality in an enterprise AI-first product is hard.
Indeed, machine learning transparency, fairness, explainability, etc. … are ill-defined concepts. How to put them into practice is still an open problem.
What does it mean at Zelros?
Because approaches for transparent and fair AI are evolving quickly, we are mobilizing an active research activity on this topic. This is the way to embed the latest and most promising methods in our product. As an example, here is an overview of the trends we are observing.
Culture
Why it matters?
Instilling fair and transparent AI is not only about regulating its utilization, or making it technically explainable. It’s also a lot about genuine organization culture, values and leadership convictions.
What does it mean at Zelros?
On top of our mission and company vision, we have at Zelros well defined core values: ambition, humility and trust. Those are the compass of all our actions, and trust is one of our guidelines helping us think about AI with the sense of our responsibilities.
Security
Why it matters?
As a user and a human being, our experience of technology is sometimes tainted by the doubt that our personal data is used to a greater extent that we would allow.
This doubt could be lifted with better transparency, but also by anonymization tools.
Consolidating users’ trust is a primary key for developing responsible AI use.
What does it mean at Zelros?
Anonymization is tricky because it is difficult to enforce without losing relevant information in the process. To overcome the performance/anonymisation trade-off requirement, we are researching new ways to provide best services while preserving anonymity, through synthetic data generation, federated learning or homomorphic encryption.
Data
Why it matters?
When discussing trustworthy AI, we often think about explainability of algorithms, transparency of purposes, or bias in data.
But what is equally important, is how you collect the data used for training. Is there consent from the data provider? Is this collection sustainable? What is the social and environmental impact of the process?
What does it mean at Zelros?
We sometimes need to crowdsource large scale data to train our computer vision or NLP models for insurance process automation. We decided to partner with Isahit, an impact sourcing platform for digital tasks. Labeling and manual data generation jobs are made by women in emerging and developing countries. It contributes to their digital inclusion, and provides them additional incomes to finance their projects, or help continue their education.
Here is an impact example of one of our recent campaign.

Want to know more about trustworthy AI, and application to financial services? Find us on our blog, twitter or linkedin.